Our faces are one of our main identifying features – not only when interacting with other people but also, nowadays, with our technology. From unlocking our phones to getting through customs at the airport – facial recognition is becoming an increasingly popular method among biometric recognition systems due to its convenience.
Despite its popularity, some issues restrict its usability, especially when used in law enforcement environments, where accuracy is of utmost importance. Mistakes and inconsistencies in the creation of the software can have serious consequences. Luckily, they are avoidable through decoding our own biases and revising our software accordingly.
Machines with Sight
In order to understand why mistakes occur, it is crucial to examine how machine vision works. Facial recognition programs are fed a main set of data called a training set as it is often done in machine learning. This data consists of a variety of different templates.
Templates are created as facial images and broken down into their most significant visual characteristics, such as eye, nose, and chin position. They are then converted into a mathematical representation which is used for reference when encountering a new face. Over time, machines can expand their repertoire and recognize more faces based on this large training set.
Equipped with this data training set, the software enables phones, computers, and robots, among other things, to detect faces for many different purposes. For example, a biometric picture is used on our ID’s and most insurance cards. But it is not only your face that holds biometric information. Despite the image, an ID often holds information on fingerprints as well. Some other identifiers are the iris or retina, voice, and even signature. Facial recognition is actually among the least accurate in this group.
Algorithmic Bias
At first glance, it might seem that machines should be inherently neutral, but generic facial recognition software actually has a problem with bias. Many of the default training sets used in this generic software are based on a disproportionate amount of pictures of white faces, especially those of males. As a consequence, these programs exhibit a significant discrepancy in accuracy between subjects with different skin tones and gender – a concept called algorithmic bias.
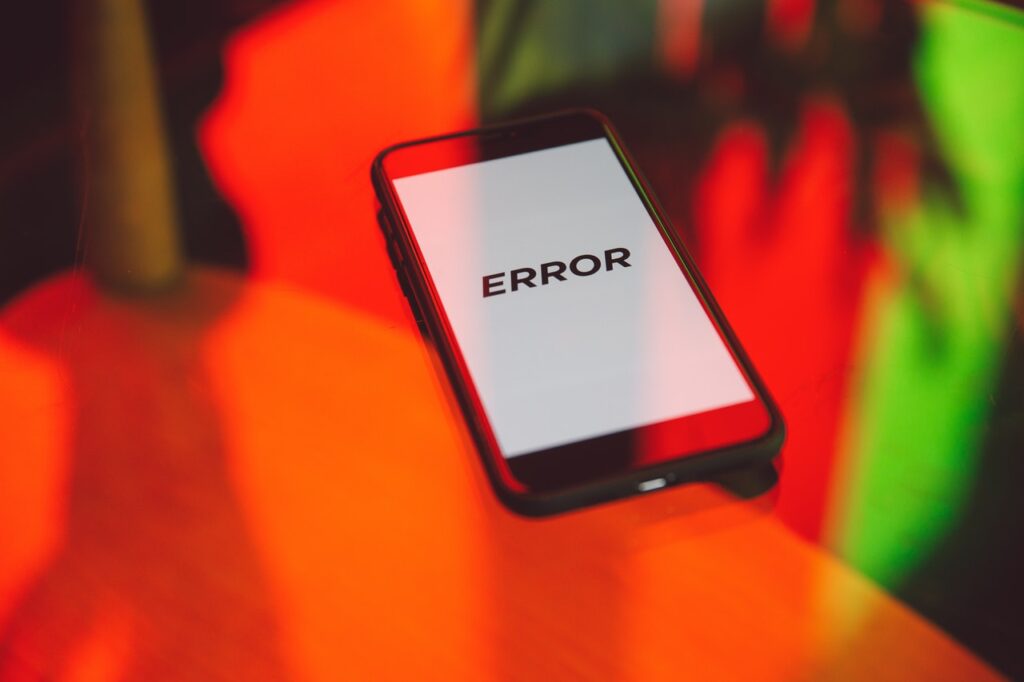
In an evaluative project, three major developers of facial recognition software were tried regarding accuracy in gender determination. For most of the subjects the gender determination was largely accurate, mainly for white males and, in one case, for black males. The evaluation shows that algorithmic bias affects mostly women of color as they were mislabeled most frequently by all three softwares. The discrepancy in error rate between white males and black women was up to 34%.
Joy Buolamwini, grad student at MIT and founder of the Algorithmic Justice League (AJL), encountered a similar problem during her studies. Her work with social robots exposed her to the fact that generic software often fails to recognize certain faces more than others. After experimenting with the software, she realized that the same generic program that could not detect her face at all, suddenly recognized her when she put on an indistinct, featureless white mask. This phenomenon travels across the globe as standard facial recognition software is easily accessible and used for different purposes throughout the world by a simple download.
Consequences
Errors in facial recognition software can be divided into two key concepts. First, there are “false negatives.” This occurs when a facial recognition system fails to recognize a face and matches it to an image within the database like it happened to Buolamwini. Second, there are “false positives,” which occurs when a face is matched with the wrong image.
While it might be funny at times to see your face mismatched to the wrong person on your phone, these errors can have severe consequences when these technologies are used in other areas. In the United States, facial recognition software is frequently used in U.S law enforcement, the job market, and for economic matters, despite its shortcomings.
There are already some cases where facial recognition software misidentified people that were accused of crimes and arrested based on a wrong match. In early 2019, for instance, an African-American man who was wrongly identified by the software used by the police had to pay 5.000 dollars in legal fees and spent ten days in prison despite being innocent.
The issue with machines is that they are programmed by humans and inherent biases can unconsciously infiltrate software. This can lead to increasing inequality and harmful social and individual practices. It reminds us that issues such as privacy are much more endangered for certain groups of people if developers do not make improving machine neutrality a priority.
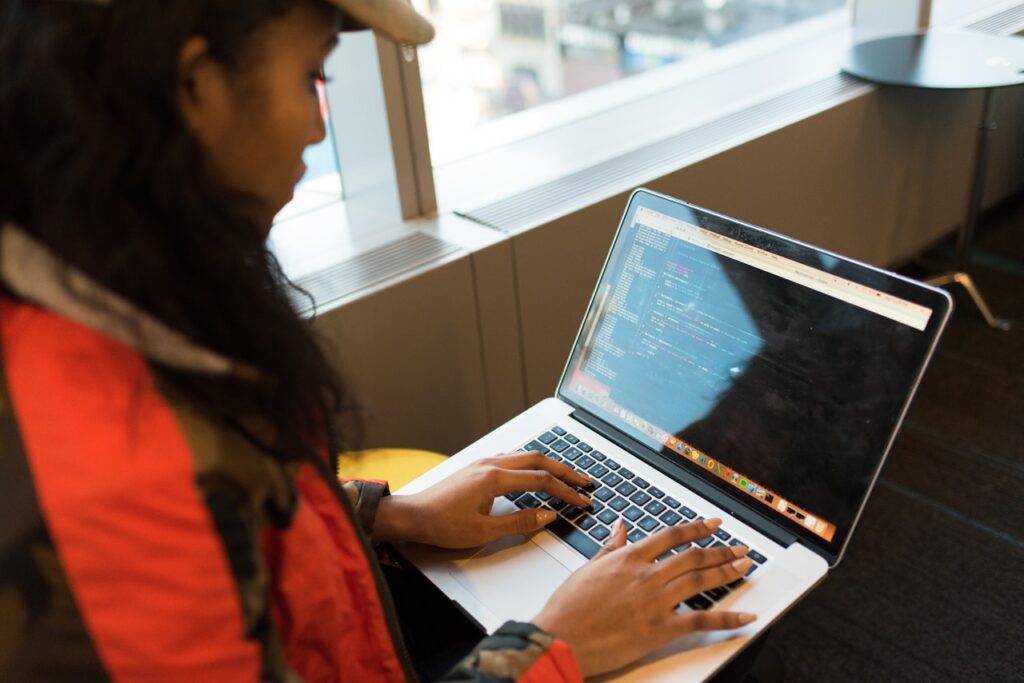
How to change coding practices
Fighting algorithmic bias may sound like a large project that affects mainly people working in information technology. However, with biometric recognition becoming more present in our daily lives and the implementation of facial recognition software in the everyday fight against crime, it is a topic that concerns us all. Machines are entirely capable of operating without or at least with a reduced bias if they are programmed with a focus on representation and diversity.
There are many ways that algorithms can be improved to be more inclusive. Accuracy in facial recognition software can be significantly enhanced by broadening training data sets for these programs. Inaccurate and potentially harmful software can also be reported to the AJL, for example, to be improved and to increase the diversity and thus accuracy standards.
But there is more that can be done: Another beneficial step is adjusting default camera settings as these are often not optimal to achieve high-quality images of every face. This results in higher error rates due to either low-quality images as input or within the training data set. Additionally, reevaluating the use of facial recognition software that does not limit the privacy, safety, and dignity of the people is necessary to avoid the abuse of this technology.
After years of work, Joy Buolamwini and the AJL have finally convinced large providers of facial recognition software to put a hold on distribution and invest more time and research into developing diverse and inclusive data training sets. Amazon, for instance, has announced a one-year moratorium on the use of their facial recognition software “Rekognition.”
While it will still be available for human rights organizations that use the software to find missing children or human trafficking victims, police must seize to use it so that a more accurate and unbiased usability can be guaranteed.
However, Buolamwini’s initial reaction to recognizing the issue with facial recognition software was much different. She says: “[…] my robot couldn’t see me. But I borrowed my roommate’s face to get the project done, submitted the assignment, and figured, you know what, somebody else will solve this problem”. Taking on the problem herself paid off. The immense progress of the project is an inspiring reminder of the power of taking action. To learn more about the initiative and its background, watch Buolamwini’s talk here:
Having a clearer understanding of the consequences of these flaws in our machines is crucial to all of us. To prevent a more substantial, underlying bias from reducing and limiting machine neutrality, taking action and raising awareness is crucial. Fighting injustice in the future begins with questioning the systems that we rely on.