“Will artificial intelligence take over the world?” That’s a question we keep hearing over and over again. Taking into consideration that in many complex situations, AI is nowhere close to “replacing” humans, we don’t have to worry about AI “taking over the world.” Well, at least for now.
At the TEDxVienna “On The Rise” event last October, we had the honor of having AI researcher Dr. Mennatallah El-Assady (“Menna”) give her first TEDx talk on explainable AI and how interactive human-AI collaboration interfaces can be used for effective problem-solving and decision-making.
Currently, Dr. El-Assady is working as a research fellow at the ETH Zurich AI Center. Before that, she was a research associate and doctoral student in the Data Analysis and Visualization group at the University of Konstanz and in the Visualization for Information Analysis lab at OntarioTech University.
Menna is not only a groundbreaking AI researcher passionate about developing AI programs but also about how to empower humans with AI agents and work together to address challenges and make informed decisions.
After her talk, I had the honor to meet and interview Menna for the TEDxVienna Magazine. We talked about what inspired her to pursue this field of research and the challenges she faced when she started to study computer science and was the only female student in a class with 130 people.
We also talked about some innovative projects she is currently working on, such as Visual Musicology and some urgent challenges AI is currently facing.
Here is the full interview with AI researcher Dr. Mennatallah El-Assady:
Were you always interested in this field of research?
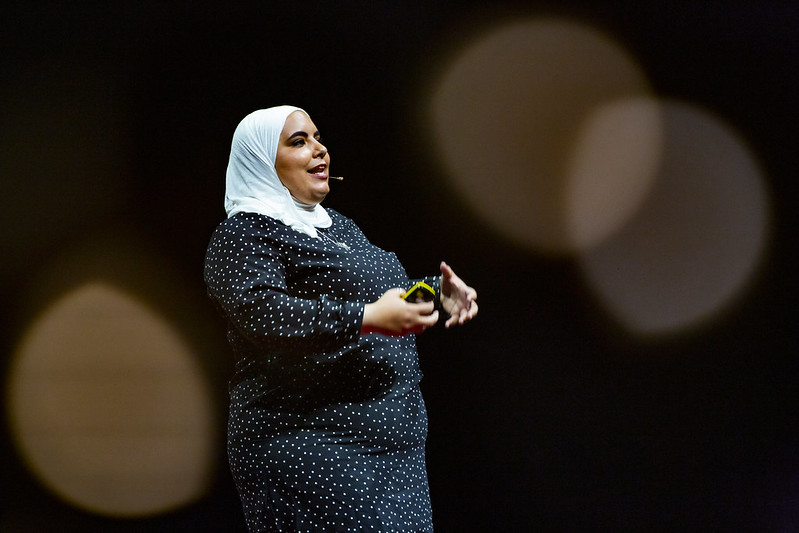
I was always interested in connecting something that you can do automatically with something you can do only as a human. But the specific connection of explaining AI models and that kind of stuff has been something that I have worked on at the very beginning of my research – but I worked more on the data analysis, graph analysis, and stuff like that. Then eventually, I figured that there are so many models that need explanations. Then I turned into explainable AI and human AI.
Was there any case while researching or doing experiments that the result was impressive or completely unexpected?
Many things are impressive, some in a very positive and some in a very negative sense. So, for example, if you look at the way we can generate language with AI – it is impressive. These modules have the capacity to learn so much data – so it is really impressive to see that data being connected. It is not surprising, but it is always exciting to see the results.
A two-year-old child will know that a cat is not just a name; it is not just a picture but will know that it has fur, is fluffy, and can purr – so all these concepts are related to this aspect of “This is a cat.” That’s something that you can expect every human to know.
If you think about it, how much do we expect a 2-year-old to know about a cat – then you will see that a 2-year-old human knows so much more about a cat than AI, which is a very simple concept: A cat.
And if you then bring some reasoning into play, for example: What will the cat do if I throw the ball? What will the cat do if I hit it- the reasoning “What would happen to the cat if I did X thing?” – is something that AI can do as well – we can train them using tools – we can sit down and write a rule that says: “If a cat does this – do that.” And we can try to infer what would happen by observing a lot of different scenarios so that we train the AI to know that if that happens, that will likely happen.
But we are still nowhere near representing this very simple concept – when we give the ball to the cat – it will run; when we give it food, it will eat, etc.
If you think about it in a more complex setting, “What would happen if a storm hits” – if you don’t have precise rules about that, AI can get it completely wrong because they only know how to infer correlations and only very simple causations. We are still nowhere near saying that we have a complex-causing model that people are working on.
In some sense, it surprises me that we can do so much with the help of AI without the AI understanding very basic concepts, but on the other hand, I am not surprised that AI can make very dumb mistakes.
So if you look at language representation and ask AI, for example, “a fish can and a fish cannot?” – the answer to both is “swim.” Because AI doesn’t understand the concept of swimming, only that it is something that is very highly correlated with the word “fish.”
How close are we to teaching complex situations to AI?
In some specific scenarios, we are very, very advanced in teaching an AI, but we are not so close to having artificial general intelligence where it can do everything. It is very narrow for specific fields.
When I think about this problem, I usually try to figure out how risky it is and how much cost is associated with whatever the AI is deciding. So, for example, if I have AI recommending products to buy on Amazon, for instance, if it recommends the wrong product to me, nothing much happens. I might buy the wrong product, and I can just return it. It doesn’t affect my life in any way since there is not a huge amount of cost associated.
If I search on Google for something and get AI to refer to me movie recommendations – all of these things don’t have a lot of costs associated with them.
But if you think about an AI for self-driving cars or weapons, all of these very high stake decisions – then it is really faithful not to get it wrong. So it depends on which situation we are configuring AI for. Then we can say that in some situations, we can use AI right away as they are right now, and they are very advanced. But these are the situations where you don’t have a lot of risks. As soon as many risks are involved, the existing AI models are not even close.
I don’t see the AIs we have at the moment making very high-risk decisions. They can assist humans in high decision-making but cannot automate high-risk decision-making right now. In specific fields, yes, but they are very narrow.
The whole spectrum is two-dimensional. One is the risk and cost. The other dimension is how well-specified the problem is. Is it something super narrow or something that has many parameters? If it is very narrow and specific, we can automate it even if it has a high risk. But if it is not, if it has many parameters and changing aspects, we cannot automate it if it is high-risk.
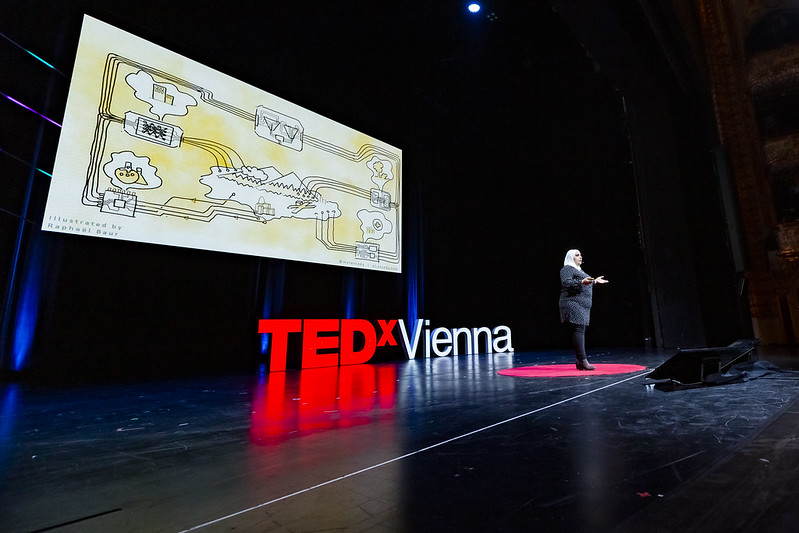
During your TEDx talk, you used climate change as the main example, explaining how both agents (humans and AI) have equal analysis. Is there any other field where AI’s role will be more important than human‘s or vice versa?
If the problem is very specific and we know the balance of the problem, then the AIs will eventually take over because we can automate it. So, for example, using images to detect specific types of skin cancer. This is something based on pictures, and we are approaching a phase where AIs are much better at doing this task than humans, as AIs make fewer mistakes.
We could automate these things at some point, but the problem with these things is that we always have to consider bias. Because for issues like skin cancer, it works well on white skin, but if you use it for darker skin tones, we don’t have enough training data for darker skin tones. Then the AI makes more mistakes. So it works well for a specific demographic but not for everyone.
Why is it that the available data is mainly for white skin tones by default?
Most of the data accessible to train AI is very Western-centric. A lot of data is coming from the US, some data is coming from Europe and Canada, and very little data is coming from other places.
The data infrastructure, the affluence of buying smartphones and sharing the data, and so on – the people collecting the data and the research funding are very Western-centric. So that’s a natural reaction to that kind of structure.
But on the other hand, we are building these tools to be used worldwide. So, it is a huge problem, for example, to train a model on western demographics, ship it to Asia or Africa, and say it can work on any type of skin. This is a problem that AI researchers need to overcome. They need to go to different places and collect data from different demographics to make it more equitable for everyone.
Can you tell us more about one of your projects called Visual Musicology?
Visual Musicology is a general research direction we started a couple of years ago with the goal of augmenting music with visuals. So that you don’t just show the music notation as it is but add some visualizations, and then you can use that on different levels of analysis.
You can use that to create an enhanced music sheet, or you can use that to create an abstract representation of music or even an abstract representation of a whole corpus like all the symphonies or all the compositions of Mozart or something like that so that you can have a fingerprint of music – that is what we are trying to do.
The Visual Musicology aspect itself, the way it started, is based on my personal history – I started learning music at a very young age, playing the flute.
When I started, I was looking for ways to learn how to read music notation, but I found it very difficult. A lot of my friends were in the same classes, and they found it super easy. Then I started coloring the notes, so A has a specific color, and I would use the colors. That made it super easy for me to read the music notes.
So I did that for years and years, playing with different colors. And then, after years of researching text data and so on, I thought, why don’t we take this idea of coloring music and apply all of the analysis we do with text and see what we can do with music? And it turns out I have dyslexia, and it is challenging for me to read these things because, in my brain, the music notes get completely twisted and turned. So I have difficulty distinguishing between different shapes. And it is something that I didn’t know as a kid.
But because of the colors, it is easier for me to read them because I can see them well – so it is a way to enhance the communication of music. When we looked into it, we saw that the way that the music notation that we use, the Western standard music notation, has developed over time. It is because, in ancient times, they needed ways for the chorus and the churches to sing, and they annotated tiny marks saying this is high, this is low, and so on.
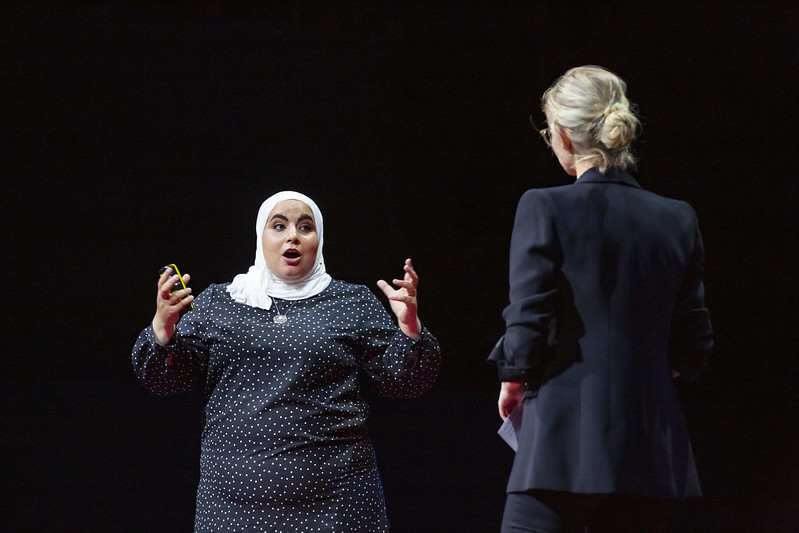
And then, if you look at the history, we have black and white dots on a line because color wasn’t available then, and they didn’t have color printing presses at the time. So this is where the story is left over. If we now sit together and think about what would be the best music representation based on all the different shapes and forms, and colors that we have, we might come up with a completely different representation that wasn’t available when history developed.
This was, for me, an interesting challenge: How do we change that? We came up with many different representations depending on what you want to do, such as harmony, melody, or rhythm, but there are many more. And the goal is not to replace the standard music annotation, but to augment it, to add to it. So you can add color to the existing notes, highlight something, or annotate it. All of these enhance the way you read music. So this is basically the whole project.
Do you take into account different types of color blindness as well?
There are different features where you can say you want a color-blind safe option, and then you get different types of color maps that are tailored to different kinds of color blindness.
Right now, the prototypes don’t support this for the music board, but one of the things that is very easy to add is to allow people to create their own colors. For example, what color should represent note C? Maybe you have your own imagination, like “C should be red,” – so you can do that.
Besides colors, did you consider something else for visualizing this?
We did consider many different things, like shapes and small representations (glyphs). The design space is endless. You can do anything and any combination. We are working on not only the design of different representations but the infrastructure to allow you to do these types of representations. So if you come up with a new design, we now have the infrastructure to take that design and make it happen so you can share your designs with others.
On what other models and systems are you working on?
We are working with political scientists on systems for conflict resolution or conflict monitoring. We are also working with medical doctors on systems to monitor the heart, other systems to analyze language, and many other systems and models.
But when I say, “we are working on them,” it is the students I am advising, so the students should get the credit, not me (laughs).
It’s also great seeing you inspire many girls and women to pursue this field of research. Was it easy for you to follow this particular field?
For me, it’s an honor to be someone who inspires them. When I started to study computer science, I was the only female and the only non-German student in a class with 130 people. And there were zero female professors as well. So I felt out of place and didn’t know whether I would continue.
The other students in my class were placing bets when I would drop out because they didn’t think I would continue because, in their eyes, I didn’t belong. I even considered dropping out and called my parents and told them, “I would be coming home soon. Expect me in 2-3 weeks”. And every 2-3 weeks, I would say to them, “In two more weeks, I will come home.” When I entered the first exams, I thought that I would fail all of the exams, but when the exam results came back and I not only passed but did really well, I thought “Well, this is an exception, maybe I just got lucky.”
I kept on thinking that maybe I would fail the next semester or the one after that, and I kept on thinking this way until I realized I was almost done with my bachelor studies and also had lots of master’s credits even before starting my master’s, so I thought maybe I should pursue my master’s. And I kept doing this until I said to myself, I know a lot about this stuff, so maybe I should do a Ph.D. (laughs)
It is great to be on the other side, be confident and share my story, but it has not been the same from day one – thinking that I would go there and conquer the world. It wasn’t like that.
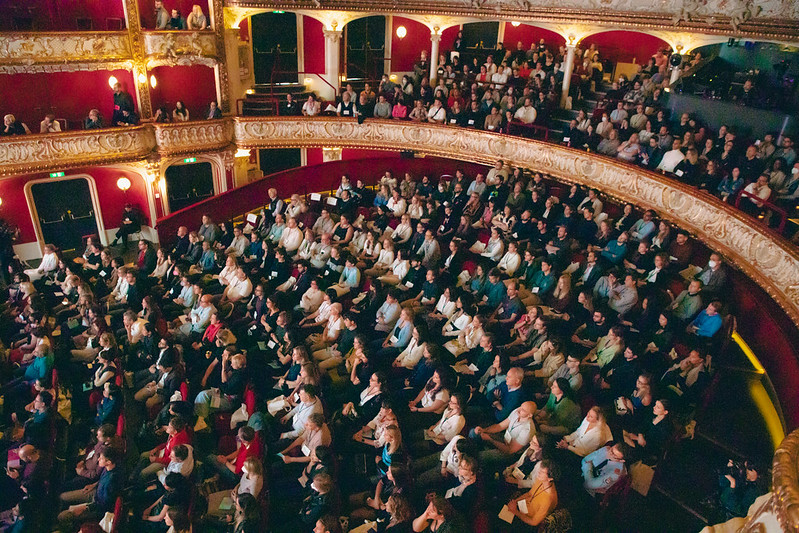
You mentioned during your TEDx talk that there are bigger AI challenges ahead. Can you please elaborate more on that and mention some urgent ones?
For me, the most urgent challenge is for many different scientific disciplines to come together and work on creating these types of systems because if we don’t develop them together to be adaptive or take into account the ethical part, and so on, we might end up with these types of systems being way too dystopian.
So to avoid having systems that might cause harm intentionally or unintentionally, we need to research how to develop these systems. And to develop these systems correctly, we need people from psychology, philosophy, social sciences, and linguistics – all these types of disciplines to come together and work together.
For me, the biggest challenge is coordinating these types of interdisciplinary research.
In terms of the good and bad things about AI, the biggest challenge is that these systems are becoming more powerful, making what we know as “truth” very muddy.
Nowadays, you can generate video, text, and all sorts of things, but you don’t know if that is the truth – the real world – or something that an AI model generated. So being able to make society, the general public, more literate about AI so they can know what to believe and what not to believe and be aware when someone is using these models for manipulation.
So training and teaching people to know these types of situations and to be able to assess them. Because a lot of times, when I talk to older folks who are not from computer science, they can fall prey to scammers who use these types of systems and a lot of auto-generated content.
That is why training the general public without having them go to computer science school is essential, and we need to do that right away.
Stay tuned for the video of her entire talk at TEDxVienna ON THE RISE, to be posted here soon!
To keep up with future updates by Dr. El-Assady and the projects she is working on, check out her personal website.